
Predictive Maintenance Solutions
We use AI for condition monitoring of your machines in order to prevent downtimes!
What we do
craftworks develops tailor-made solutions for any kind of use case to optimize the performance of your machines. Our predictive maintenance solutions help to reduce equipment failures, machine downtimes and cost. Insights based on artificial intelligence and recommendations make maintenance more efficient for operators. It gives them better understanding for downtime causes and insight that can help prevent such incidents in future.
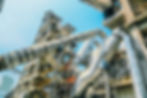
SUCCESS STORY
District heating network
Our client, a utility company owns one of the biggest district heating networks in Europe, consisting of thousands of converter stations. In these plants, water is being cooled before it is delivered to their customers.
Challenge
Unplanned downtimes used to occur on a daily basis. Operators had to constantly replan scheduled maintenance to be available for the repair of broken machines, which makes it a highly inefficient process.
Solution
Our AI powered predictive maintenance solution does much more than common cmms software. Based on historical data, our machine learning algorithm predicts potential downtimes seven days in advance. Moreover, the solution finds unknown correlations between certain data sets and downtimes, which helps to understand what causes those downtimes.
Result
Based on these insights, more efficient maintenance schedules can be set in order to avoid those downtimes. The knowledge of root causes can be used for fine tuning settings. This starts as a recommendation system and can gradually transfer to automated parameter setting.
The Process
In a first step, the team of the utility company and craftworks were sitting together in order to find out which problems should be tackled and to set goals. In this case, it was the inefficiency in the maintenance work that should be addressed.
After the use case was defined, the teams from both companies checked if there was enough quality data and got the first understanding of processes, parameters, and their values. In certain cases, it was necessary to use additional sensors from a trusted craftworks partner company.
In a next step, relations and patterns in sensor-, machine-, and process data were recognized and a machine learning model and architecture were developed.
At the end of the first phase, a simple software prototype was reviewed verifying the recommendations and identifying enhancements.
More Success Stories

Resource efficiency with Predictive Maintenance
Predicting gearbox failures for cost efficient processes and lower emissions
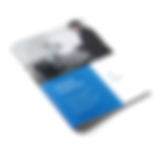
Predictions of robot failures in spot welding application
A solution that suggests corresponding instructions for actions in order to decrease operational downtime by approximately 30%.
​

Predicting disruptions in advance
Accurate predictions between seven days and one month in advance
How you can work with us…
Consultancy
You would like to be advised on the basic setup of an industrial AI approach in your company? You need guidance in how machine learning can help you make your processes more efficient and cost effective? Through tailor made workshops we will help you find the right approach for your company.
​
Co-Development
You already have a functional team in place, but you are short on manpower or specific experience? Our team is used to working closely with our customers on new solutions and facilitates through knowledge sharing.
​
​
​
​
Full Service
You are looking for a trusted partner to develop a robust customised software solution to your specific needs and requirements? Well, you found us!
​
​
​
​
​
​
… and how we make you independent

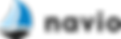
Would you like to independently create your own models but need a way to ship machine learning straight to production? With navio you can deploy, manage and monitor machine learning models. Seamlessly integrate with your existing workflow via the API. Simply focus on training machine learning models and navio takes care of the rest with a one-click deployment, saving you time and money.
​
A selection of our Industrial AI clients


































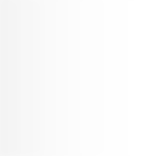
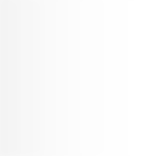
“
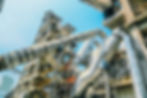